What is AI analytics?
AI analytics refers to the use of artificial intelligence technologies to analyze data and extract insights in a way that is beyond the capabilities of traditional data analysis methods.
By leveraging machine learning algorithms, AI analytics can identify patterns, predict outcomes, and provide personalized recommendations by processing vast amounts of data at incredible speeds.
This advanced form of analytics enables businesses to make more informed decisions, optimize operations, and enhance customer experiences by delivering content that is tailored to individual preferences and behaviors.
AI analytics is the application of artificial intelligence to process and analyze data. It involves using machine learning, natural language processing, and data mining techniques to interpret data and make predictions or recommendations.
AI personalization leverages these analytics to deliver a more individualized experience to users. By examining past interactions, purchase history, and even social media activity, AI systems can curate content, suggest products, and even adjust user interfaces to better suit individual needs.
This level of customization is not only beneficial for enhancing user satisfaction but also for increasing engagement and loyalty, as consumers tend to favor services that resonate with their personal tastes and preferences.
AI analytics is a specialized area within data analytics, which is part of the broader fields of business analytics and business intelligence. These fields all focus on transforming data into actionable insights.
Leveraging AI for personalization involves utilizing machine learning algorithms to sift through vast amounts of data, identifying patterns and preferences unique to individual users.
This enables businesses to tailor their offerings and communications to match the specific needs and desires of their customers, enhancing the user experience and fostering loyalty.
By analyzing past behaviors, purchase history, and even real-time interactions, AI-driven systems can predict future preferences and deliver recommendations that are increasingly precise and relevant.
Traditional analytics relies on basic statistical analysis techniques like regression analysis, hypothesis testing, and descriptive statistics. These methods provide insights into data patterns and relationships but are limited in scope and complexity.
Traditional approaches involve time-consuming manual data processing in Microsoft Excel spreadsheets and manual reporting and visualization. Before AI, analytics relied on simpler forecasting models and techniques, such as time-series analysis and linear regression, which yielded limited predictive capabilities and needed labor-intensive processes.
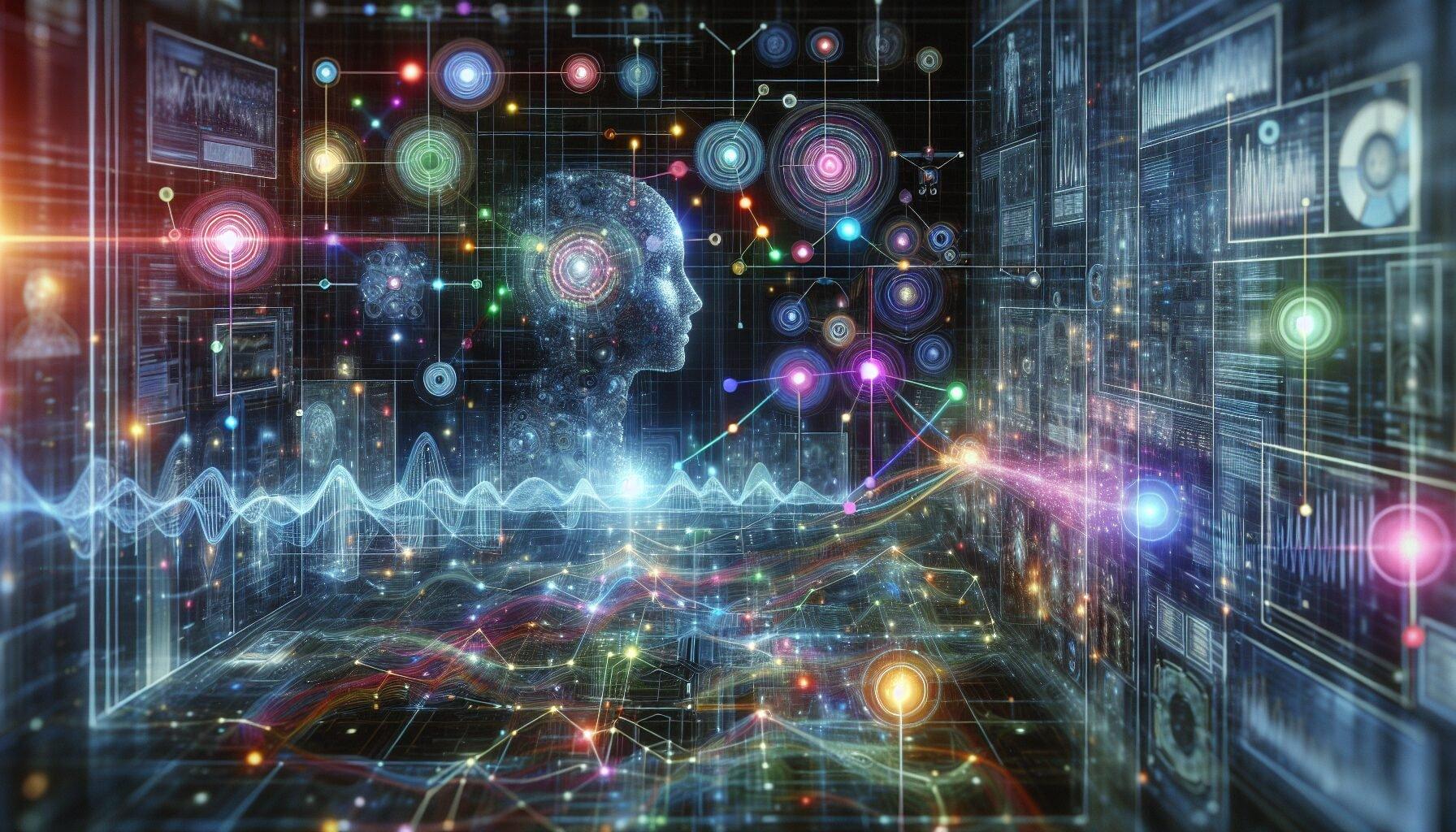
With the advent of AI personalization, the landscape of data analytics has been transformed. Advanced algorithms and machine learning techniques now allow for more sophisticated pattern recognition, enabling predictions that adapt to changing data in real time.
This shift not only enhances the accuracy of forecasts but also significantly reduces the time and resources required for data analysis. AI-driven tools can now learn from new data as it becomes available, continuously refining their models for more precise personalization and decision-making support.
The implementation of AI in business analytics has become an imperative for large organizations looking to maximize competitive advantage. AI’s ability to quickly process large volumes of data, identify patterns, and generate predictive insights offers a significant competitive edge.
This enables businesses to make data-driven decisions that improve various key performance indicators (KPIs), reduce costs, and improve business outcomes.
The latest AI News + Insights
AI personalization stands at the forefront of this technological revolution, transforming the customer experience into something far more tailored and engaging. By leveraging sophisticated algorithms, companies can now anticipate customer needs, customize recommendations, and deliver dynamic content that resonates with individual preferences and behaviors.
This level of customization not only enhances user satisfaction but also fosters brand loyalty and increases the likelihood of repeat business, solidifying AI’s role as an invaluable asset in the modern marketplace. Discover expertly curated insights and news on AI, cloud computing, and more in the weekly Think Newsletter.
Types of AI analytics
Understanding the different types of AI analytics is crucial for businesses looking to leverage artificial intelligence for personalized customer experiences.
Predictive analytics, for instance, uses AI algorithms to analyze historical data and predict future behavior, enabling companies to anticipate customer needs and preferences. Meanwhile, prescriptive analytics goes a step further by not only forecasting outcomes but also suggesting actions to achieve desired results.
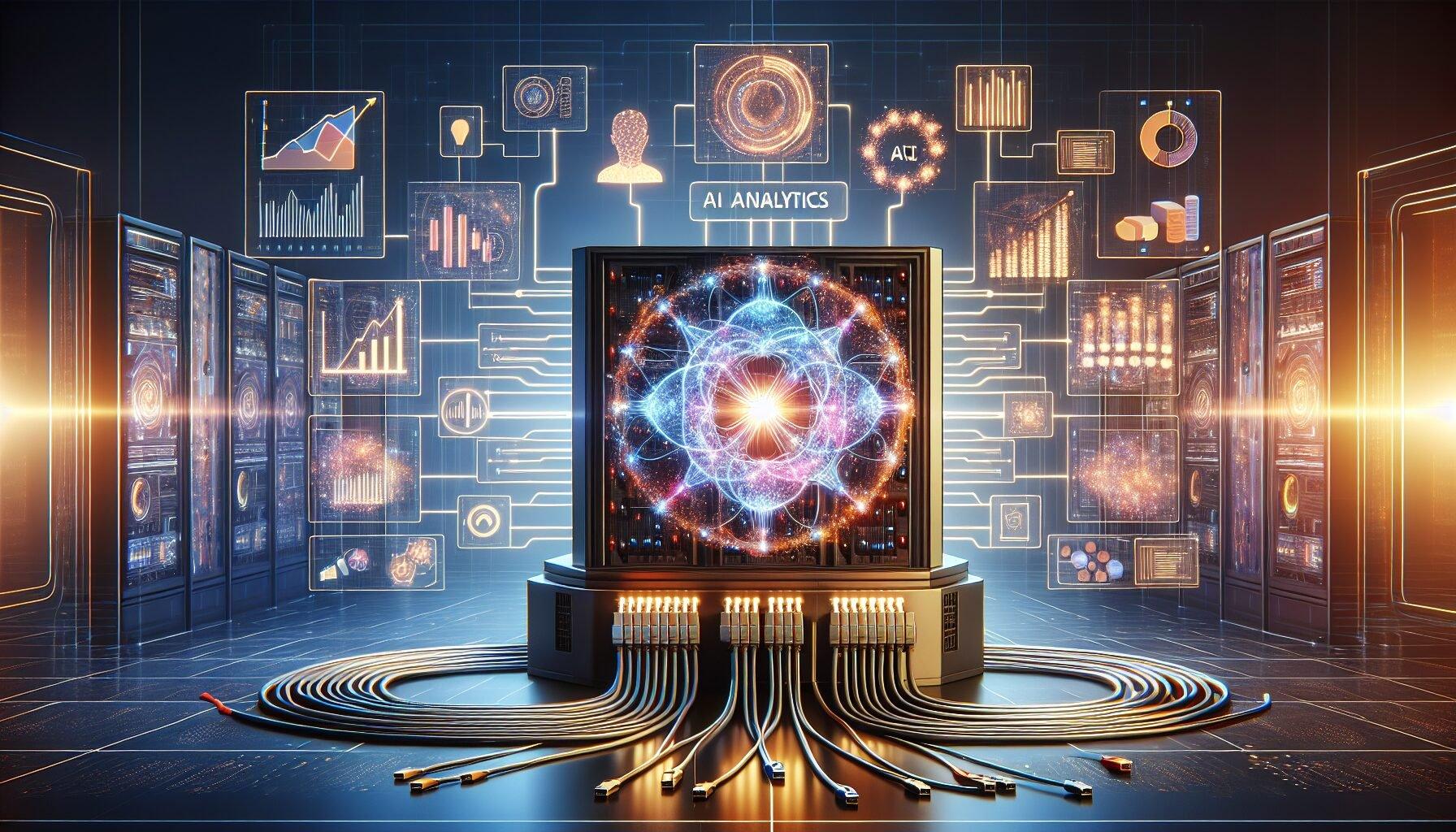
By integrating these AI analytics into their strategies, businesses can create highly tailored interactions that resonate with individual customers, driving engagement and satisfaction. There are four main types of data analytics, and AI can be used to enhance all four.
Descriptive analytics: “What happened?”
Predictive analytics: “What is likely to happen?” AI excels at predicting future behavior by analyzing patterns and trends from past data. By leveraging machine learning algorithms, AI can forecast customer actions, anticipate needs, and preemptively offer solutions or products. This proactive approach not only streamlines the customer experience but also increases the likelihood of conversion and customer loyalty.
AI-powered descriptive analytics provides a clear and comprehensive understanding of past performance. With machine learning algorithms and natural language processing (NLP), AI can sift through large volumes of structured and unstructured data to identify patterns, trends, and correlations. Consider a retailer deploying AI algorithms to analyze customer data to uncover insights about purchasing trends and preferences.
By leveraging these insights, businesses can tailor their marketing strategies to deliver highly personalized experiences to their customers. For instance, an AI system might send targeted product recommendations or personalized discounts to individual customers based on their previous shopping behavior and predicted interests.
This level of personalization not only enhances customer satisfaction by making interactions feel more relevant and engaging, but it also drives increased loyalty and potentially higher sales for the business.
For instance, an AI system can process millions of sales records and social media trends to identify which products are most popular during certain seasons or which items are frequently bought together.
This customer behavior analysis can then be visualized on dashboards, showing sales performance over time, customer segmentation based on purchasing habits, and the effectiveness of marketing campaigns.
By providing a detailed and comprehensive view of past customer experiences, AI-powered descriptive analytics helps retailers make data-driven decisions to optimize inventory management, tailor marketing strategies, and enhance customer satisfaction.
Diagnostic analytics: “Why did it happen?”
Building upon the insights from descriptive analytics, AI-driven diagnostic analytics delve deeper, seeking to understand the underlying causes of observed patterns and outcomes. By leveraging advanced algorithms and machine learning techniques, these systems can sift through complex data to identify correlations and root causes.
This level of analysis is crucial for retailers as it enables them to pinpoint specific factors that influence customer behavior, sales trends, and operational inefficiencies, allowing for more informed strategic decision-making and proactive problem-solving.
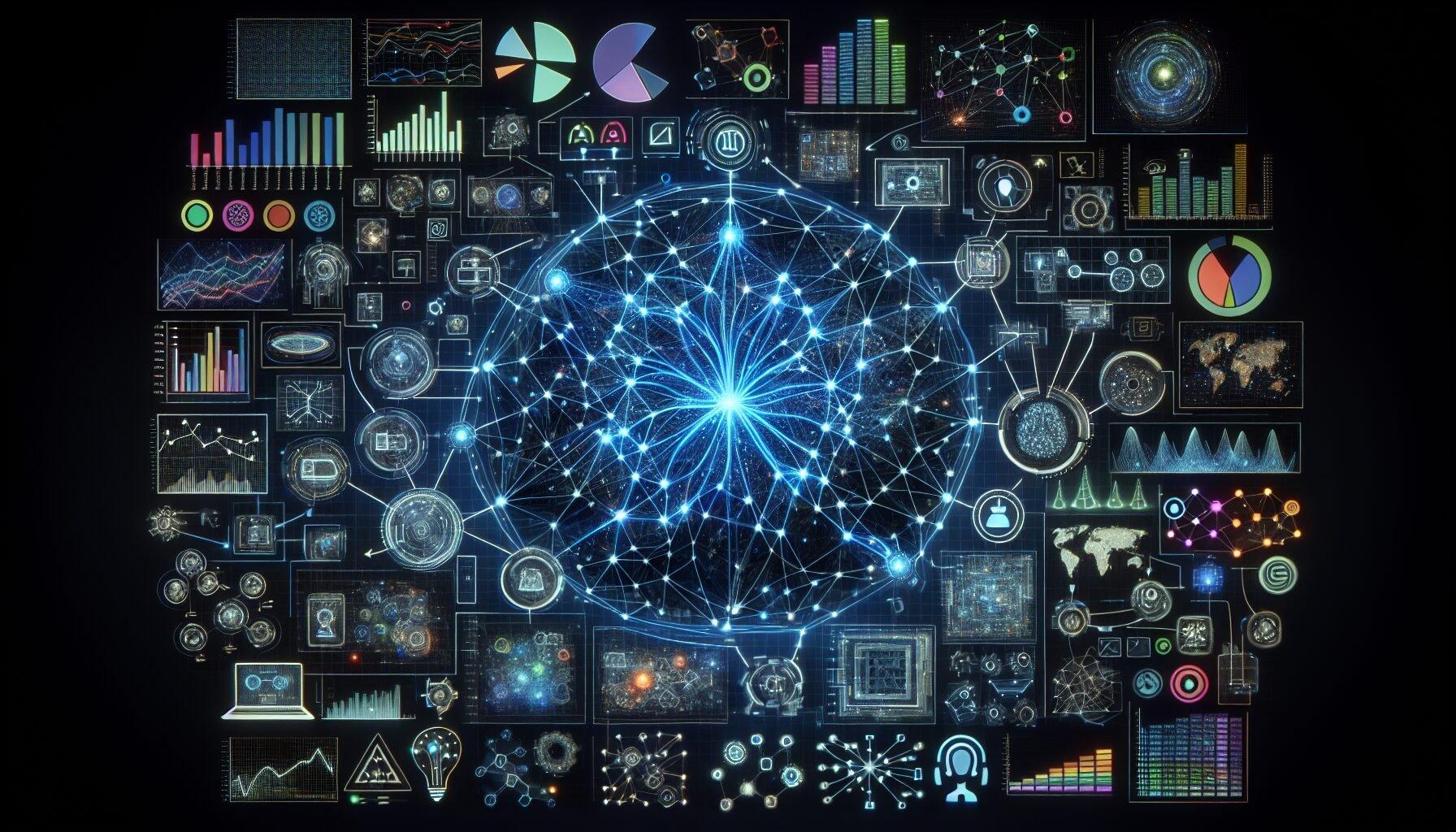
AI improves diagnostic analytics by rapidly identifying underlying causes and correlations within complex datasets, enabling more accurate and timely problem-solving. An example of how AI improves diagnostic analytics can be found in healthcare. AI algorithms analyze patient data, including medical histories, lab results, and imaging scans.
These algorithms identify the root causes of diseases and conditions more accurately and quickly than traditional methods. For instance, AI can detect patterns in radiology images that indicate early stages of diseases like cancer, providing doctors with valuable insights that aid in accurate diagnosis and personalized treatment plans.
Predictive analytics: “What might happen next?”
Predictive analytics harnesses the power of AI to forecast future events based on historical data, which is invaluable in personalizing customer experiences. By analyzing past consumer behavior, AI can predict future purchasing patterns, allowing businesses to tailor their marketing efforts and product recommendations to individual preferences.
This level of personalization not only enhances the customer journey but also increases engagement and loyalty, as customers feel understood and valued by brands that seem to “know” them and their needs. AI enhances predictive analytics by using advanced algorithms to analyze historical data and identify patterns, enabling more accurate forecasts of future trends and outcomes.
In financial services, machine learning models analyze historical market data and economic indicators to forecast stock prices and investment risks, helping investors make informed decisions and manage their portfolios more effectively.
Prescriptive analytics: “What should we do next?”
AI personalization extends the capabilities of prescriptive analytics by tailoring recommendations to individual user preferences and behaviors. In the realm of e-commerce, for example, AI algorithms can study a customer’s past purchases, search history, and even the time spent on certain product pages to suggest items that align closely with their tastes.
This level of customization not only enhances the shopping experience for the consumer but also boosts sales and customer loyalty for businesses, as users feel understood and catered to on a personal level.
Analysts can take advantage of AI to not only analyze data but to provide actionable recommendations for better decision-making processes and suggest the best course of action for various scenarios.
An example of AI used in prescriptive analytics is in supply chain management, where AI systems analyze data on inventory levels, demand forecasts, and shipping conditions. These systems recommend optimal order quantities and delivery schedules, thereby minimizing costs and improving efficiency.
Decoding AI: Weekly News Roundup
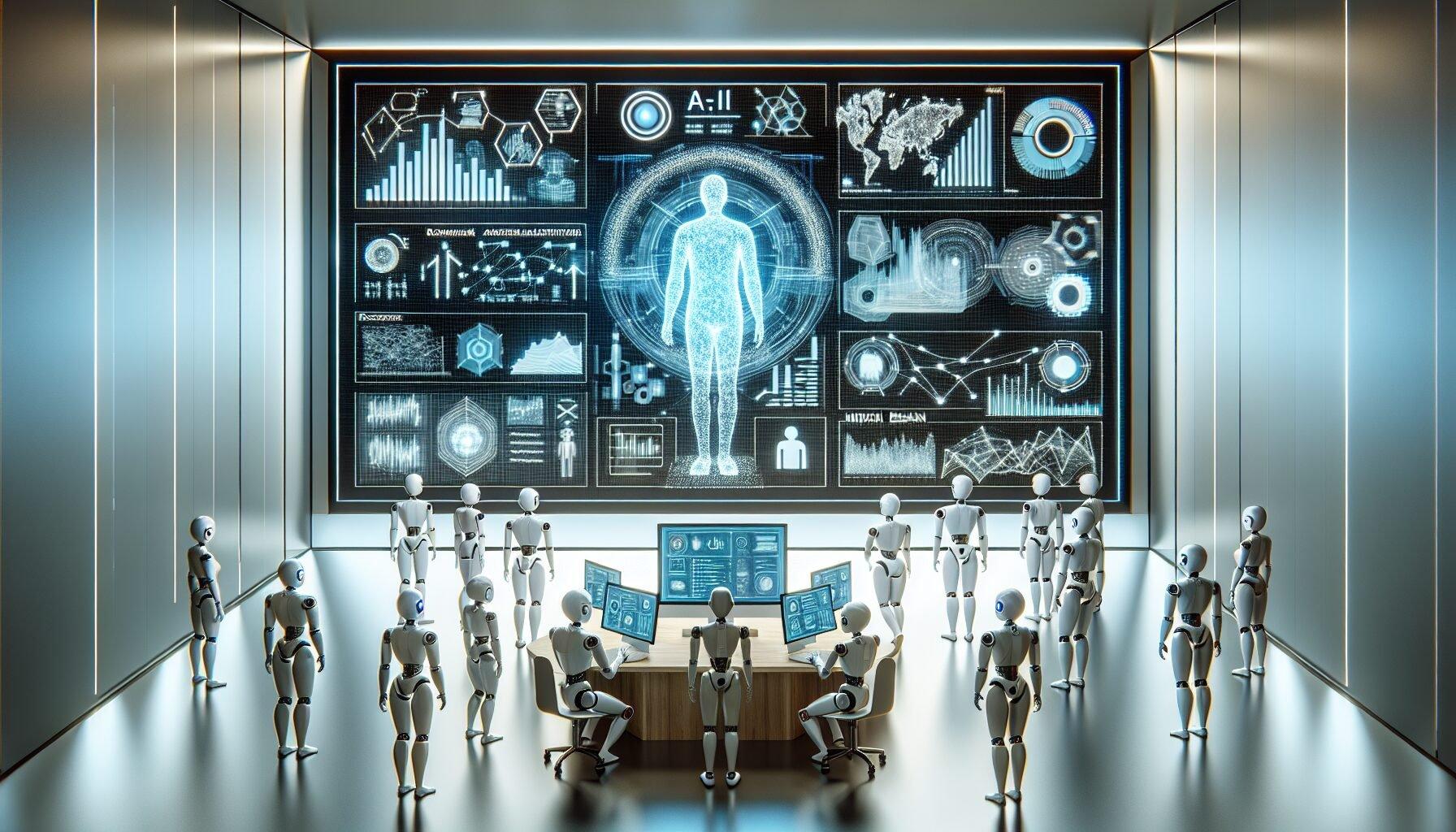
In the realm of marketing, AI personalization is revolutionizing the way businesses interact with their customers. By leveraging machine learning algorithms, companies can now tailor their advertising and content to suit individual preferences, creating a more engaging and relevant experience for each user.
This not only enhances customer satisfaction but also significantly boosts conversion rates and fosters brand loyalty. As AI continues to learn from user behavior, these personalized experiences become increasingly sophisticated, ensuring that consumers are met with content that resonates with their unique interests and needs.
Join our world-class panel of engineers, researchers, product leaders, and more as they cut through the AI noise to bring you the latest in AI news and insights.
Watch the latest podcast episodes
How AI analytics works
In this rapidly evolving landscape, AI personalization stands as a beacon of innovation, offering a tailored experience that is as unique as the individual engaging with it.
By harnessing the power of machine learning algorithms and data analytics, AI systems can predict user preferences and deliver content that not only meets but anticipates the needs of the consumer.
This transformative approach to user interaction not only enhances the user experience but also drives engagement, creating a symbiotic relationship between technology and personal preference. AI-powered analytics typically follows these steps:
Defining the problem
To effectively leverage AI personalization, it is crucial to pinpoint the specific issues that consumers face, which can range from navigational difficulties within an app to finding relevant content on a sprawling website.
Once the problem is clearly defined, AI algorithms can be tailored to address these challenges by analyzing user behavior and engagement patterns.
This data-driven approach allows for the creation of a dynamic and responsive environment that anticipates user needs and preferences, delivering a seamless and intuitive experience that feels uniquely tailored to each individual.
Practitioners must first identify what the model is expected to predict to select the most appropriate approach. Different models are tailored for specific use cases, and choosing the right model and data is crucial for achieving optimal results quickly and cost-effectively.
Data collection and preprocessing
Once the model’s purpose is clearly defined, the next critical step is data collection and preprocessing. This involves gathering relevant data from various sources and ensuring it is clean, structured, and suitable for training the AI.
Preprocessing may include tasks such as normalizing data, handling missing values, and encoding categorical variables, all of which are essential to ensure the AI can learn effectively from the data and provide personalized experiences.
Workflows begin with data scientists or data analysts gathering relevant data from both internal data sources and external third-party providers. The accuracy and effectiveness of the models depend on the quality and quantity of the data collected.
Once gathered, the data is preprocessed to clean, transform, and prepare it for analysis. This step involves addressing missing values, removing duplicates, standardizing formats, and encoding categorical variables to ensure that the data is consistent and ready for modeling.
Descriptive and predictive analytics
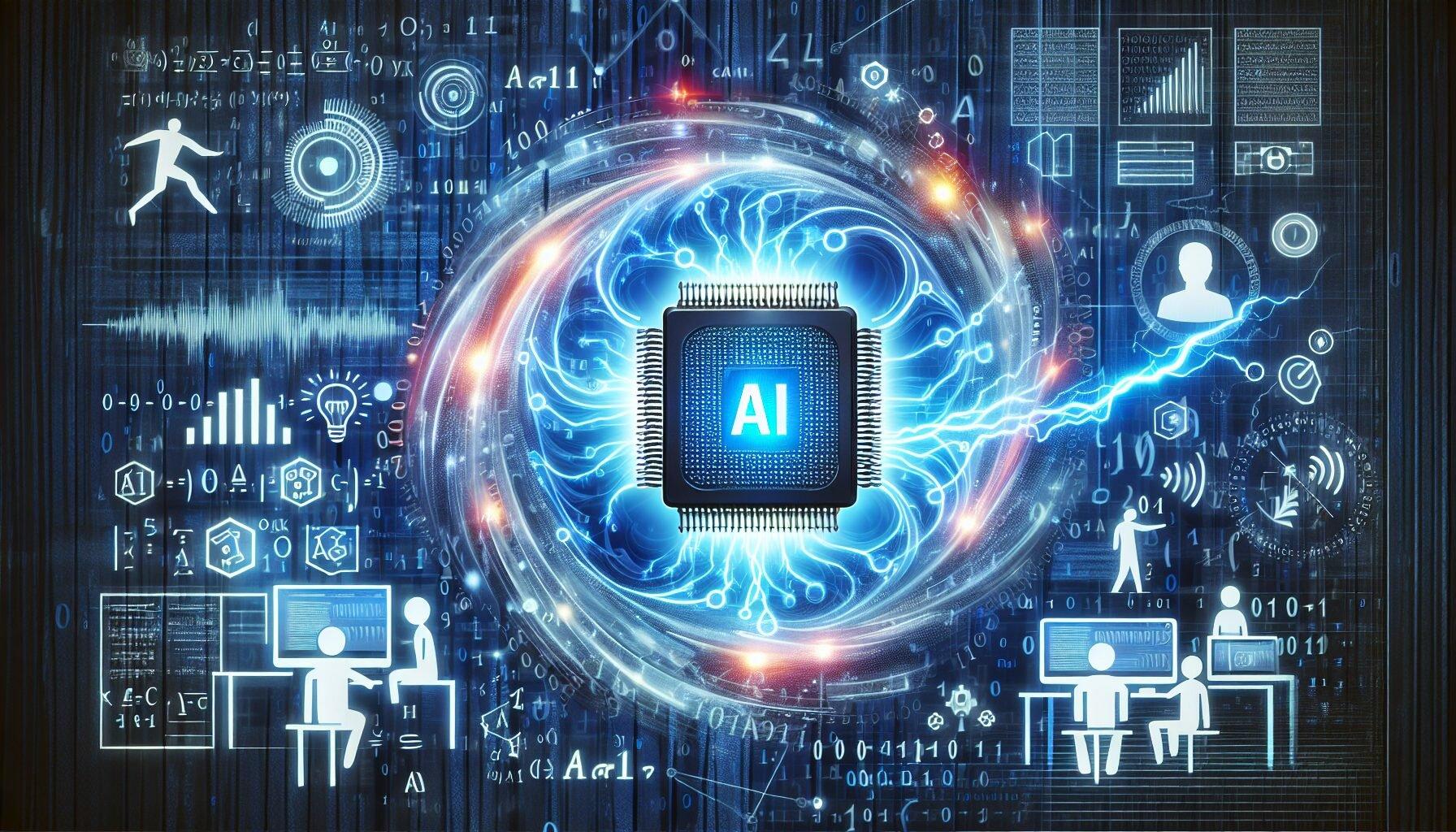
Once the data is primed for action, descriptive analytics come into play, offering a detailed interpretation of past behaviors and trends. This analysis provides a foundational understanding of the data at hand, which is crucial for identifying patterns that can inform future strategies.
Predictive analytics then takes the baton, utilizing statistical models and machine learning algorithms to forecast potential outcomes. By integrating these insights, AI personalization systems can tailor experiences to individual users, enhancing engagement and satisfaction through highly relevant content and recommendations.
Before applying prescriptive analytics, analysts generally perform descriptive analytics to review past performance and predictive analytics to project future outcomes.
Descriptive analytics involves summarizing and visualizing data to gain insights into historical trends and patterns, while predictive analytics uses statistical and machine learning models to forecast future events or behaviors.
Prescriptive modeling
Prescriptive modeling takes predictive analytics a step further by not only forecasting future trends but also suggesting actions that can be taken to capitalize on these predictions. This level of analytics employs complex algorithms and machine learning techniques to provide decision-makers with actionable recommendations.
By analyzing the potential outcomes of different decisions, prescriptive modeling can optimize processes and strategies to achieve the best possible results, making it a powerful tool for businesses looking to leverage AI personalization to its fullest extent.
Prescriptive analytics involves constructing mathematical models and optimization algorithms to recommend business decisions aimed at achieving the best possible outcomes.
These models consider various factors such as constraints, objectives, uncertainties, and tradeoffs, building on insights from descriptive and predictive analyses to suggest how an organization should respond to different scenarios.
Deployment
The deployment of AI personalization requires careful integration into existing systems and workflows to ensure seamless user experiences. It involves the strategic placement of personalized elements within digital interfaces, whether it be on websites, mobile applications, or even in email marketing campaigns.
This step is critical as it not only brings the analytical predictions to life but also allows for real-time adaptation and learning from user interactions, thereby continuously refining the personalization engine for more accurate and effective results.
After evaluation, the models are integrated into operational systems or applications to provide real-time predictions and recommendations. This might involve incorporating the models into existing software systems, APIs, or dashboards to automate decision-making processes or offer prescriptive insights to users, facilitating a more seamless use of insights.
Monitoring and refinement
As AI personalization technologies mature, continuous monitoring and refinement become essential to maintaining their effectiveness. This involves regularly analyzing performance data to identify any deviations from expected outcomes and recalibrating the models accordingly.
By doing so, businesses can ensure that their AI-driven recommendations remain relevant and accurate, thereby enhancing user satisfaction and engagement over time. Additionally, this iterative process helps in adapting to changing user behaviors and preferences, keeping the personalization efforts aligned with current trends and needs.
Continuous monitoring and maintenance of the models are necessary to ensure their ongoing effectiveness and relevance. This includes tracking model performance, updating with new data, and periodically retraining and refining the models to adapt to changing conditions or evolving data patterns.
History of AI analytics
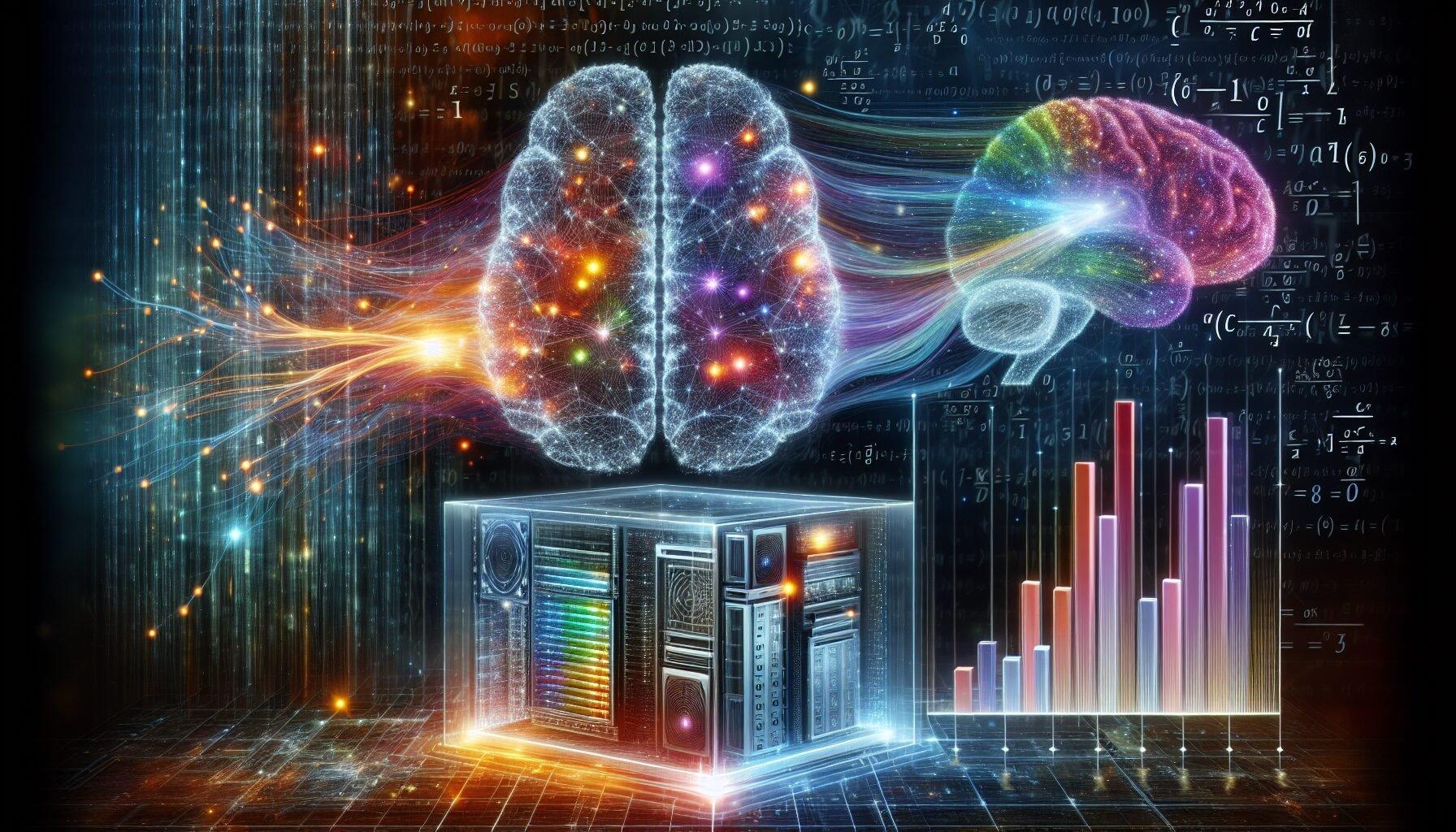
As the field of AI analytics has evolved, it has become increasingly intertwined with the concept of personalization. The historical trajectory of AI has seen it move from simple rule-based algorithms to complex machine learning and deep learning models capable of delivering highly individualized experiences.
This shift has been driven by the explosion of data and advancements in computational power, allowing for the processing and analysis of vast data sets to identify patterns and preferences unique to each user. As a result, AI personalization has emerged as a critical tool in enhancing user engagement and satisfaction across various industries.
The history of AI analytics traces the evolution of both artificial intelligence and data science, marking significant milestones in both technology and methodology. This history culminates in a true golden age of AI analytics that characterizes our current moment.
Early foundations (1950s-1960s)
The birth of AI personalization can be traced back to these early foundations, as researchers began to explore the potential for machines to not only process vast amounts of data but to learn from it and make decisions.
Pioneering efforts in the mid-20th century laid the groundwork for what would become machine learning and, eventually, deep learning—techniques at the heart of today’s AI personalization.
It was during this era that the concept of “adaptive systems,” which could tailor outputs to individual users based on data-driven insights, started to take shape, foreshadowing the highly personalized digital experiences we see now.
The concept of AI began to take shape, with Alan Turing’s work on computing and intelligence laying the theoretical groundwork. Early AI research focused on symbolic AI and rule-based systems, which were the precursors to modern data analytics techniques.
Growth of machine learning (1970s-1980s)
As the field of AI evolved, the advent of the internet and the exponential increase in data availability paved the way for more sophisticated machine learning algorithms. During the 1990s and 2000s, AI researchers began to harness the power of neural networks, which mimicked the human brain’s ability to learn from experience.
This shift marked the beginning of deep learning, a subset of machine learning that has since become integral to the development of personalized AI applications, revolutionizing the way we interact with technology by enabling systems to learn and adapt to individual user preferences and behaviors.
The advent of machine learning introduced statistical methods to AI, allowing systems to learn from data and improve over time. Neural networks and expert systems emerged, enabling more complex data analysis and reasoning capabilities.
Data explosion and early AI tools (1990s)
As the digital age progressed into the 21st century, AI personalization began to take a more defined shape, leveraging the vast amounts of data generated by users across various platforms. Sophisticated algorithms started to analyze this data, identifying patterns and preferences unique to each individual.
This led to the development of personalized experiences, where AI systems could predict user needs and tailor content, recommendations, and services in real-time, transforming the way users interact with technology on a deeply individual level.
The rise of the internet and digital data has led to an explosion in the volume of data available for analysis. Early AI analytics tools began to integrate with databases and data warehousing systems.
Advancements in algorithms and big data (2000s)
As AI technology evolved, the focus shifted towards leveraging this vast data to offer personalized experiences. Machine learning algorithms, fed with enormous datasets, began to identify patterns and preferences unique to individual users.
This marked the advent of AI personalization, where services could be tailored to meet the specific needs and desires of each person, transforming the landscape of customer interaction and engagement across various industries.
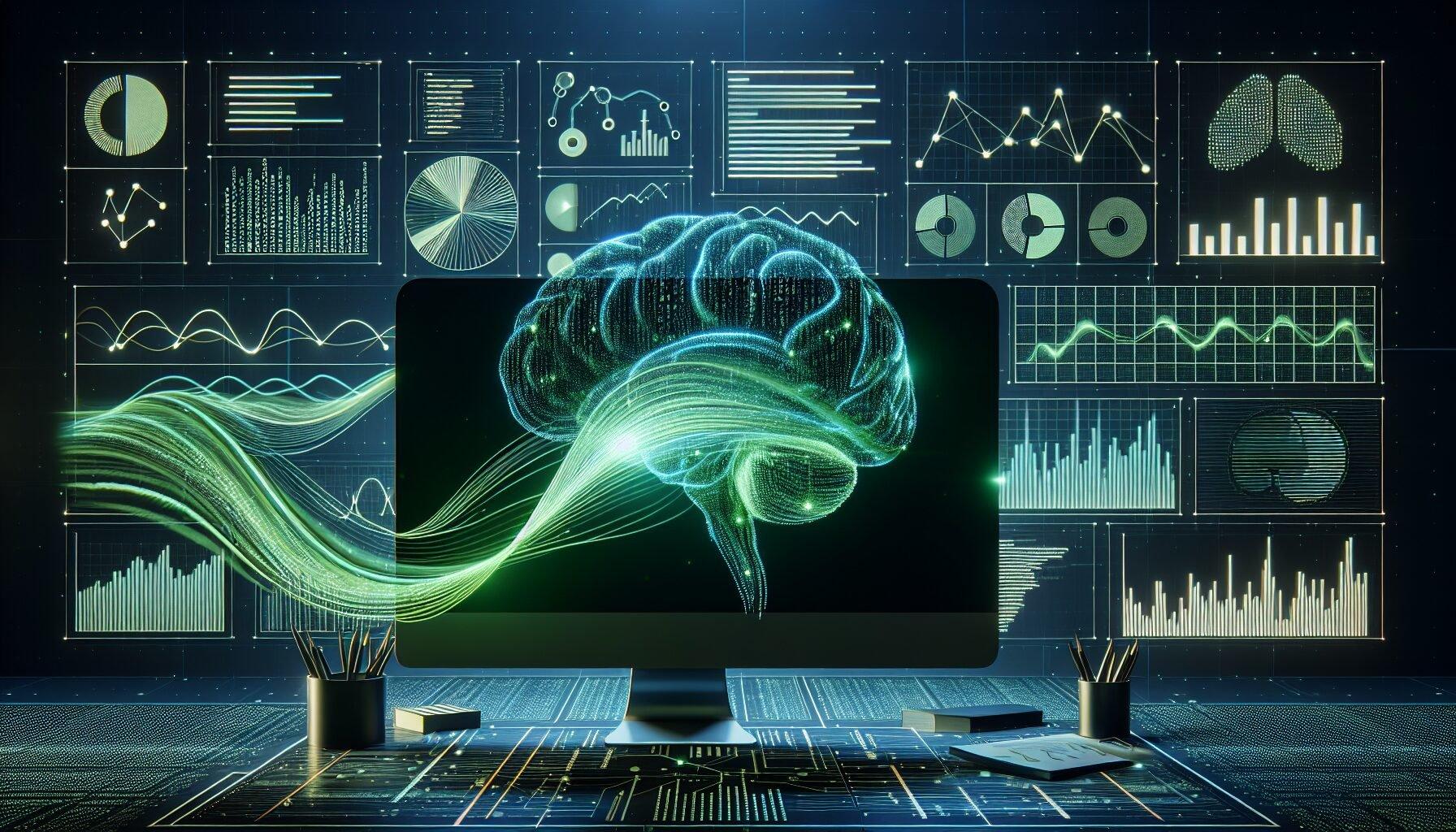
Significant advancements in algorithms and computing power led to the development of more sophisticated AI models. The concept of “big data” emerged, emphasizing the need for advanced analytics solutions to handle vast amounts of information contained in large datasets.
Deep learning and modern AI analytics (2010s-Present)
As we entered the era of deep learning and modern AI analytics, the potential for personalization has become more pronounced than ever. With the advent of neural networks capable of understanding and predicting human behavior, businesses and service providers have been able to offer highly individualized experiences to their customers.
This level of personalization has revolutionized marketing strategies, user experience design, and customer service, making interactions more engaging and tailored to the unique preferences and needs of each individual.
The resurgence of deep learning, powered by neural networks with many layers, revolutionized AI analytics platforms. Techniques such as NLP and computer vision became prominent, allowing for more advanced and nuanced analysis of data.
AI analytics now encompasses a wide range of applications. The integration of AI with cloud computing and IoT continues to expand its capabilities and applications across various industries.
As the technology matures, AI personalization is becoming increasingly sophisticated, offering tailored experiences to users like never before. By leveraging vast amounts of data and powerful predictive algorithms, AI can anticipate user preferences and behaviors, delivering content, recommendations, and services that are uniquely suited to individual needs.
This level of customization is transforming industries, from e-commerce, where personalized shopping experiences can significantly boost conversion rates, to healthcare, where AI-driven personalization can lead to more accurate diagnoses and treatments tailored to the patient’s genetic makeup and lifestyle.
Generative AI, popularized in apps such as ChatGPT, is poised to significantly impact analytics by enhancing its various aspects. For example, synthetic data creation can augment existing datasets and inform simulated scenarios.
Generative AI can also streamline and improve the process of analysis, making it more user-friendly by automating repetitive tasks, generating automated reports, creating dynamic data visualizations, and other experience improvements.